Is your digital hub primed for generative AI?
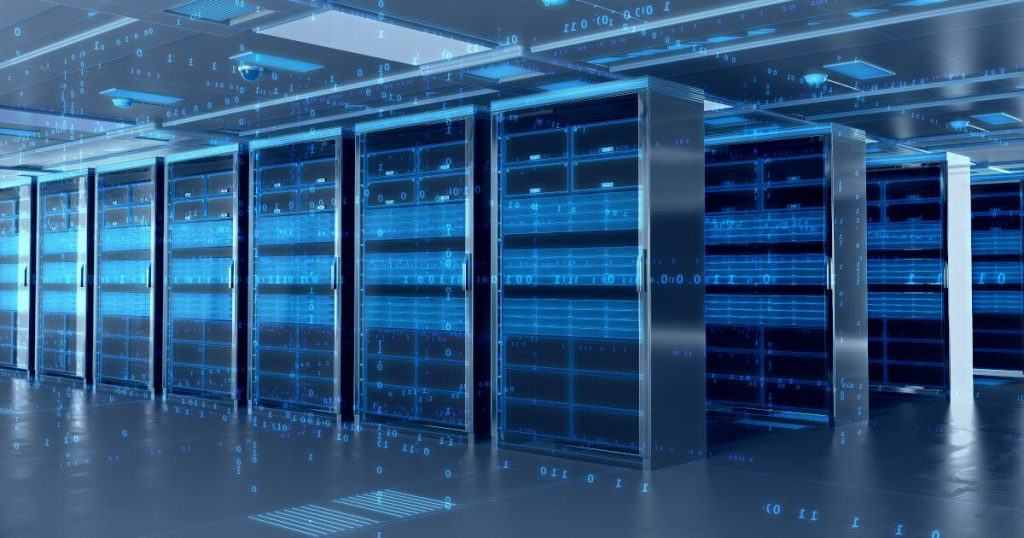
The craving for generative AI is skyrocketing, exerting a strain on the underpinnings of data centers. Generative AI, adept at generating text, imagery, and other forms of media in reaction to cues, is on the brink of being widely adopted by companies as they discover innovative applications for the technology.
The enthusiasm around generative AI is unabated,” says Frances Karamouzis, an analyst at Gartner. “Businesses are trying to gauge the extent of their financial commitment towards generative AI solutions, which ones are worth the investment, the ideal moment to get started, and how to counterbalance the risks associated with this nascent technology.”
Bloomberg Intelligence foresees that the generative AI market will grow at a staggering rate of 42% per annum over the forthcoming decade, from $40 billion in 2022 to $1.3 trillion. Generative AI has the potential to aid IT teams in numerous ways, including software code writing, networking script creation, troubleshooting, issue resolution, process automation, training, onboarding, documentation creation, knowledge management system development, and project management and planning.
It also has the capacity to revolutionize other segments of business, such as call centers, customer service, virtual assistants, data analytics, content creation, design, development, and predictive maintenance, to name just a few.
But can the existing data center infrastructures endure the escalating workload created by generative AI?
The repercussions of generative AI on computational requirements There’s no doubt that generative AI will become a key component of most companies’ data strategies. Networking and IT leaders should already be ensuring that their IT infrastructures and teams are prepared for the forthcoming shifts. As they design and deploy applications that integrate generative AI, how will that influence the demand for computational power and other resources?
“The demand on today’s data centers will rise, and will bring about a drastic transformation in the future appearances of data centers and their associated technology,” says Brian Lewis, the managing director at consulting firm KPMG.
Generative AI applications create substantial demand for computational power in two phases: the training of the large language models (LLMs) that constitute the backbone of generative AI systems, and the subsequent application operation with these trained LLMs, says Raul Martynek, CEO of DataBank, a data center operator.
“Training the LLMs necessitates dense computing in the form of neural networks, wherein billions of language or image examples are fed into a neural network system and continuously refined until the system ‘recognizes’ them on par with a human,” Martynek explains.
Neural networks require extremely dense high-performance computing (HPC) clusters of GPU processors operating non-stop for months, or possibly years, Martynek says. “They operate more efficiently on dedicated infrastructure located close to the proprietary datasets used for training,” he explains.
The secondary phase involves the “inference process” or the actual utilization of these applications to make inquiries and return data results. “In this operational phase, it requires a more geographically dispersed infrastructure that can scale rapidly and provide access to the applications with lower latency—as users querying the information will want a swift response for the anticipated use cases.”
This will necessitate data centers in numerous locations as opposed to the centralized public cloud model that presently supports most applications, Martynek says. In this phase, the demand for data center computational power will remain high, he says, “but in comparison to the initial phase, such demand is spread across more data centers.”
The push of generative AI for liquid cooling Networking and IT leaders need to consider the impact generative AI will have on server density and its implications on cooling requirements, power demands, sustainability initiatives, and so forth.
“It’s not merely density, but the duty cycle of how frequently and how much those servers are being used at peak load,” says Francis Sideco, a principal analyst at Tirias Research. “Companies like NVIDIA, AMD, and Intel are trying to boost performance with each generation of AI silicon while keeping power and thermal under control with each iteration.”
Regardless of these efforts, power budgets are still on the rise, Sideco says. “With the workloads escalating at such a rapid pace, especially with GenAI, we are bound to hit a wall at some point.”
Server density “doesn’t have to climb like we observed with blade technology and virtual hosts,” Lewis adds. “Technical innovations like non-silicon chips, graphics processing units (GPUs), quantum computing, and hardware-aware, model-based software development will be able to extract more from existing hardware.”
The industry has already been dabbling with innovative liquid cooling techniques that are more efficient than air, as well as sustainability in diverse locations such as Microsoft’s Project Natick, an undersea data center, Lewis says.
“Traditional air cooling techniques, such as fans, ducts, vents, and air-conditioning systems, are inadequate for meeting the cooling demands of high-performance computing hardware such as GPUs,” Lewis explains. “Therefore, alternative cooling technologies like liquid cooling are gaining traction.”
Liquid cooling involves the circulation of coolants like water or other fluids through heat exchangers to absorb the heat generated by computer components, Lewis explains. “Liquid cooling is more energy-efficient than traditional air cooling, as liquids have higher thermal conductivity than air, which enables better and more efficient heat transfer.”
The designs of new data centers will need to satisfy higher cooling requirements and power demands, meaning future data centers will have to depend on new cooling methods such as rear chilled doors, water to the chip or immersion technologies to strike the right balance between power, cooling, and sustainability, Martynek explains.
Data center operators are already introducing advancements in liquid cooling, Martynek says. For instance, DataBank employs a new ColdLogik Dx Rear Door cooling solution from QCooling at its facility in Atlanta that houses the Georgia Tech Supercomputer.
“We foresee a significant rise in water to the door and water to the chip cooling technologies, especially as future generations of GPUs will consume even more power,” Martynek predicts. “The demand for more computational space and power stemming from generative AI adoption will undoubtedly accelerate the quest for more efficiencies in power consumption and cooling.”
The implications of Gen AI on power requirements It may become more common for data center operators to construct their own power substations, Martynek speculates. “Demands on the electric grid due to demand and the transition to renewable power sources are creating more uncertainty around power supply, and new data center project schedules are heavily influenced by the utility company’s workload and its capabilities to handle the power needs of new facilities,” he says.
A reliable and scalable power source will increasingly be a priority for data center operators, both to keep up with the demand for power generated by HPC clusters and to overcome the timelines and limitations of utilities, Martynek speculates.
DataBank is rolling out a new data center design standard dubbed the Universal Data Hall Design (UDHD), which features a slab floor with perimeter air cooling and greater spacing between cabinets that is ideal for hyperscale cloud deployments and can be deployed quickly, Marty